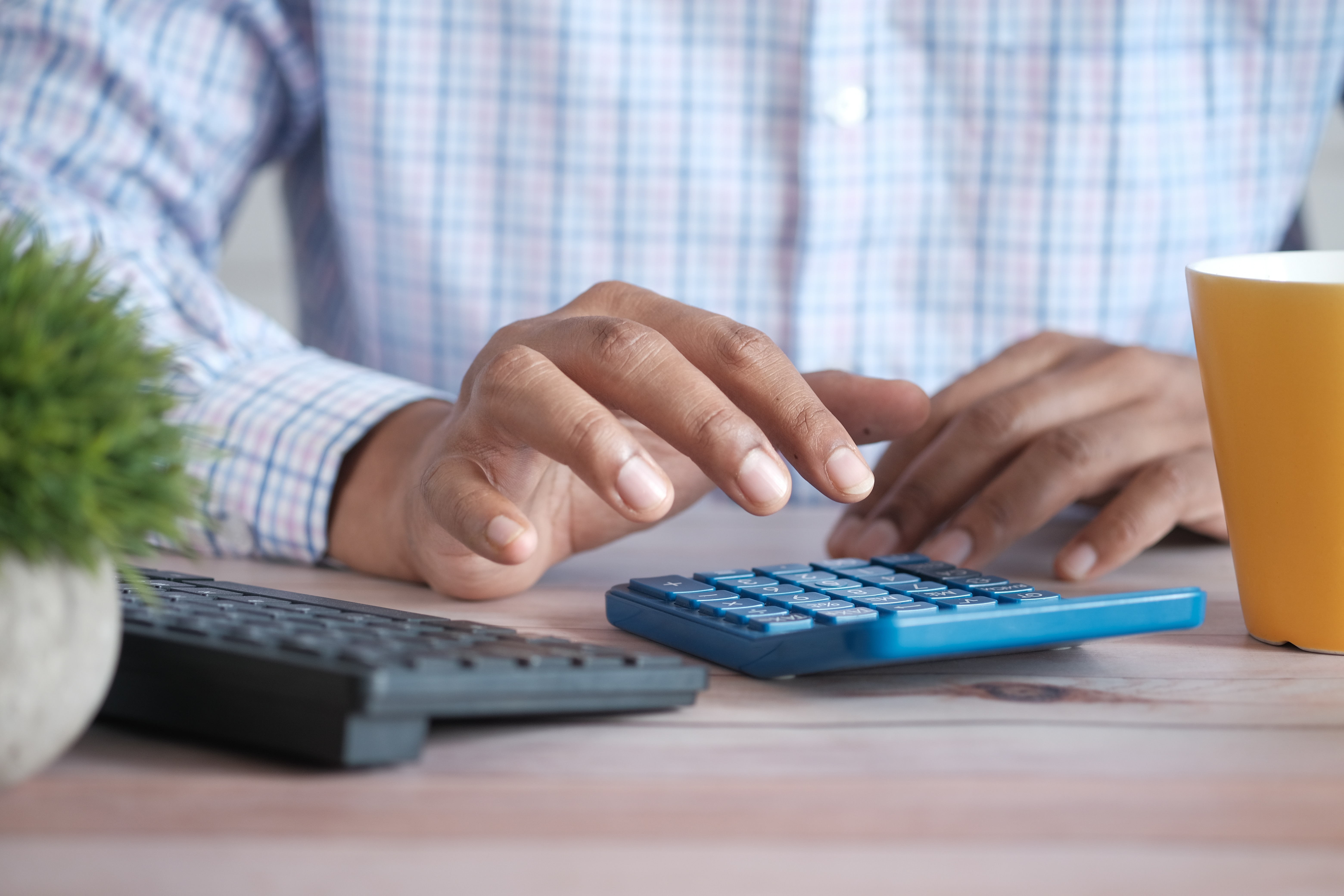
Why Cloud workload costs are often underestimated
by Peter Shi
Throughout my experience in Cloud Economics consulting, I have encountered many cases in which the run-cost of cloud workloads are underestimated by customers.
When these underestimated cloud expenses are incorporated into the budget, it often leads to a scenario where the cloud budget is depleted before the close of the fiscal year. This causes many organizations to lose momentum for cloud adoption and necessitates a reactive scramble to address the issue through cost optimization.
This reactive response often manifests as Finance reaching out to Cloud Engineering, requesting a cost optimization investigation and seeking to understand why cloud expenses exceeded budget. However, these efforts often encounter challenges such as:
- Cloud Engineering is preoccupied with meeting deadlines for other essential projects and hesitates to prioritize cost optimization over the needs of other business stakeholders.
- Cloud Engineering may be averse to making changes to customer-facing environments that can yield cost savings, citing previous negative experiences.
- Some Cloud Engineering teams lack expertise in cost optimization methods and tools.
- The project team who created the budget and deployed the infrastructure may no longer be around or available.
- It is difficult to understand who and what is driving the cost overrun due to a lack of a clear account or tagging strategy
As interesting as it would be to deep dive into why optimization efforts are typically slowed, this blog post will focus on why cloud workload costs are underestimated in the first place.
Hopefully in reading this post you'll be more aware of what drives cloud costs to be underestimated.
Pressure and incentive to produce a low cost estimate
Pressure and incentive to produce low cost estimates is a common driver of underestimates. Cases where I've seen this include:
- A cloud vendor is responding to a request for proposal and is compelled to submit a low-cost bid in order to improve chances of winning the contract.
- A cloud partner is looking to justify migration to cloud, and wants to show the cost savings as part of the business case for the move.
- A team wants to have a project approved so estimates are intentionally kept within some financial approval threshold.
In all three scenarios, while the final figures may be technically feasible, aggressive assumptions around the model sets a very high bar for execution which makes it difficult to achieve.
Inaccurate assumptions
Whether due to incentive to produce a low cost estimate or inexperience in budgeting cloud costs, I've seen inaccurate assumptions lead to insufficient cloud budget. Here are two examples.
Non-production environment assumptions
Non-production environments can typically be sized smaller than production and its compute and database resources can be turned off outside of work hours (saving 50% to 70%).
This can lead cost modellers to estimate that dev/test will cost around 30% of production.
Based on observing hundreds of live environments, although dev/test can cost at little as 10% of production (after optimization efforts), more typically their costs hover around 50% to 80% of production cost. Prior to optimization efforts, non-production environments tend to:
- Be kept on 24/7. Switching off non-production requires new tooling, process, and change management
- Be sized to match production. This is both for testing convenience and adherence to legacy policies that do not prioritise cost efficiency
Reservation assumptions
'Reservations' that provide discount in exchange for commitment exist on all major cloud providers. Examples include AWS and Azure Savings Plans, AWS and Azure RIs, and GCP CUDs.
In modelling the impact of reservations, three key variables drive the final cost result.
Assumption | Cost model with aggressive or overly-optimistic assumptions | Average enterprise environment |
---|---|---|
Commitment term and discount rate | 3-year reservation saving 50% to 70% | Mix of 1-year and 3-year reservations saving 35% on average |
Reservation coverage. i.e., how much of the resources will be covered, and thus, discounted by a reservation | 100% coverage | 60% for compute and 20% for databases |
Reservation utilization. i.e., how much of the reservation will actually be used | 100% utilization | 90% to 95% utilization |
Final saving | 50% to 70% lower vs. on-demand costs | 20% to 25% lower vs. on-demand costs |
Forgetting to include key cost items
Another way that cost estimates can be too low is by forgetting to include certain cost line-items. Here are some cost line items I've seen customers forget to include:
- Development and testing
- Data Transfer, which can be within regions, between regions, out to internet, and from/to on-premises data centers
- VPC (Virtual Private Cloud), logging (e.g. CloudWatch), and load balancing
- Support costs
To avoid underestimates and cloud budget overruns consider:
- Checking if any of the above 'lessons learned' apply in your case and adjust the model/budget accordingly
- Use a cloud pricing guide and checklist
- Add some cost contingency to the budget
- Have an independent expert review the cost model, and/or
- Apply 'FinOps' practices and ensure engineering teams that generate the spend are aware of the budget
Hopefully this post will help you avoid future cases of cloud cost underestimates and potential cost overruns.
> See other blogs
> Contact us